开课单位--纽约大学
1
2
3
4
5
6
7
8
9
10

Density Modeling of Images using a Generalized Normalization Transformation[利用广义归一化变换的图像密度建模]
Johannes Ballé(纽约大学) We introduce a parametric nonlinear transformation that is well-suited for Gaussianizing data from natural images. The data are linearly transformed, ...
热度:18
Johannes Ballé(纽约大学) We introduce a parametric nonlinear transformation that is well-suited for Gaussianizing data from natural images. The data are linearly transformed, ...
热度:18

Discussion of Erik Sudderth's talk: NPB Hype or Hope?[讨论Erik Sudderth的演讲:NPB炒作还是希望?]
Yann LeCun(纽约大学) Discussion of Erik Sudderth's talk: NPB Hype or Hope?
热度:20
Yann LeCun(纽约大学) Discussion of Erik Sudderth's talk: NPB Hype or Hope?
热度:20

Energy-based models & Learning for Invariant Image Recognition[基于能量的不变图像识别模型与学习]
Yann LeCun(纽约大学) Energy-based models & Learning for Invariant Image Recognition
热度:21
Yann LeCun(纽约大学) Energy-based models & Learning for Invariant Image Recognition
热度:21

Acquisition of Lexical Knowledge from N Grams[从N个语法中获取词汇知识]
Satoshi Sekine(纽约大学) Acquisition of Lexical Knowledge from N Grams
热度:23
Satoshi Sekine(纽约大学) Acquisition of Lexical Knowledge from N Grams
热度:23

Unsupervised Learning of Disease Progression Models[疾病进展模型的无监督学习]
David Sontag(纽约大学) Chronic diseases, such as Alzheimer's Disease, Diabetes, and Chronic Obstructive Pulmonary Disease, usually progress slowly over a long period of ...
热度:24
David Sontag(纽约大学) Chronic diseases, such as Alzheimer's Disease, Diabetes, and Chronic Obstructive Pulmonary Disease, usually progress slowly over a long period of ...
热度:24
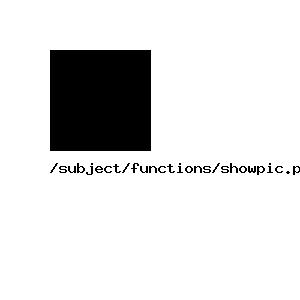
Convolutional Neural Networks and Computer Vision[卷积神经网络与计算机视觉]
Rob Fergus(纽约大学) This talk will review Convolutional Neural Network models and the tremendous impact they have made on Computer Vision problems in the last few years. ...
热度:22
Rob Fergus(纽约大学) This talk will review Convolutional Neural Network models and the tremendous impact they have made on Computer Vision problems in the last few years. ...
热度:22

Adversarial Autoencoder and Multi-Task Semi-Supervised Learning for Multi-Stage Process[多阶段过程的对抗式自动编码器和多任务半监督学习]
Andre Mendes da Silva(纽约大学) Adversarial Autoencoder and Multi-Task Semi-Supervised Learning for Multi-Stage Process
热度:11
Andre Mendes da Silva(纽约大学) Adversarial Autoencoder and Multi-Task Semi-Supervised Learning for Multi-Stage Process
热度:11

Natural RLDM: Optimal and Subptimal Control in Brain and Behavior[自然RLDM:大脑和行为的最佳和次最佳控制]
Nathaniel Daw(纽约大学) Approaches to reinforcement learning and statistical decision theory from artificial intelligence offer appealing frameworks for understanding how bio...
热度:48
Nathaniel Daw(纽约大学) Approaches to reinforcement learning and statistical decision theory from artificial intelligence offer appealing frameworks for understanding how bio...
热度:48

An Empirical Analysis of Sponsored Search Performance in Search Engine Advertising[搜索引擎广告赞助搜索绩效的实证分析 ]
Anindya Ghose(纽约大学) An Empirical Analysis of Sponsored Search Performance in Search Engine Advertising
热度:46
Anindya Ghose(纽约大学) An Empirical Analysis of Sponsored Search Performance in Search Engine Advertising
热度:46
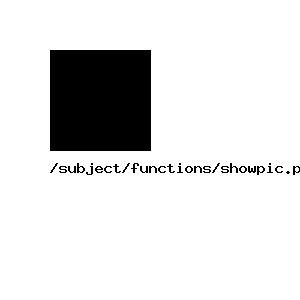
10. DARPA LAGR Program: Learning Applied to Long-Range Vision using a Collision-Free Navigation Platform[DARPA LAGR程序:学习运用避碰导航平台的长期愿景]
Pierre Sermanet, Chris Crudele, Ayse Erkan, Yann LeCun, Urs Muller, Raia Hadsell, Marco Scoffier, Matthew Koichi Grimes, Jan Ben(纽约大学) New York University uses machine learning to extend the vision of the DARPA LAGR robots.
热度:26
Pierre Sermanet, Chris Crudele, Ayse Erkan, Yann LeCun, Urs Muller, Raia Hadsell, Marco Scoffier, Matthew Koichi Grimes, Jan Ben(纽约大学) New York University uses machine learning to extend the vision of the DARPA LAGR robots.
热度:26