开课单位--多伦多大学
51
52
53
54
55
56
57
58
59
60

BoltzRank: Learning to Maximize Expected Ranking Gain[BoltzRank:学习最大化预期排名增益]
Maksims Volkovs(多伦多大学) Ranking a set of retrieved documents according to their relevance to a query is a popular problem in information retrieval. Methods that learn rankin...
热度:140
Maksims Volkovs(多伦多大学) Ranking a set of retrieved documents according to their relevance to a query is a popular problem in information retrieval. Methods that learn rankin...
热度:140

Non-Linear Matrix Factorization with Gaussian Processes[高斯过程的非线性矩阵分解]
Raquel Urtasun(多伦多大学) A popular approach to collaborative filtering is matrix factorization. In this paper we consider the "probabilistic matrix factorization" an...
热度:113
Raquel Urtasun(多伦多大学) A popular approach to collaborative filtering is matrix factorization. In this paper we consider the "probabilistic matrix factorization" an...
热度:113
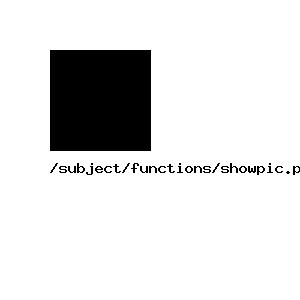
A Simpler Unified Analysis of Budget Perceptrons[预算感知的一个更简单的统一分析]
Ilya Sutskever(多伦多大学) The kernel Perceptron is an appealing online learning algorithm that has a drawback: whenever it makes an error it must increase its support set, whic...
热度:38
Ilya Sutskever(多伦多大学) The kernel Perceptron is an appealing online learning algorithm that has a drawback: whenever it makes an error it must increase its support set, whic...
热度:38
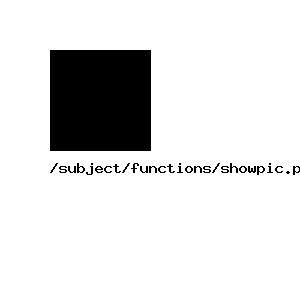
Tractable Nonparametric Bayesian Inference in Poisson Processes with Gaussian Process Intensities[具有高斯过程强度的泊松过程中的可跟踪非参数贝叶斯推断]
Ryan Prescott Adams(多伦多大学) The inhomogeneous Poisson process is a point process that has varying intensity across its domain (usually time or space). For nonparametric Bayesian ...
热度:99
Ryan Prescott Adams(多伦多大学) The inhomogeneous Poisson process is a point process that has varying intensity across its domain (usually time or space). For nonparametric Bayesian ...
热度:99

Archipelago: Nonparametric Bayesian Semi-Supervised Learning[Archipelago:非参数贝叶斯半监督学习]
Ryan Prescott Adams(多伦多大学) Semi-supervised learning (SSL), is classification where additional unlabeled data can be used to improve accuracy. Generative approaches are appealing...
热度:98
Ryan Prescott Adams(多伦多大学) Semi-supervised learning (SSL), is classification where additional unlabeled data can be used to improve accuracy. Generative approaches are appealing...
热度:98

Topologically-Constrained Latent Variable Models[拓扑约束潜变量模型]
Raquel Urtasun(多伦多大学) In dimensionality reduction approaches, the data are typically embedded in a Euclidean latent space. However for some data sets this is inappropriate....
热度:63
Raquel Urtasun(多伦多大学) In dimensionality reduction approaches, the data are typically embedded in a Euclidean latent space. However for some data sets this is inappropriate....
热度:63
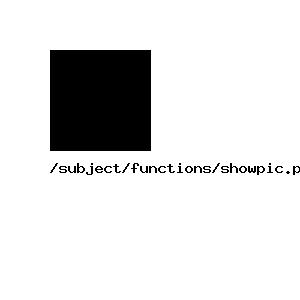
Training Restricted Boltzmann Machines using Approximations to the Likelihood Gradient[训练受限玻尔兹曼机器使用逼近似然梯度的近似]
Tijmen Tieleman(多伦多大学) A new algorithm for training Restricted Boltzmann Machines is introduced. The algorithm, named Persistent Contrastive Divergence, is different from th...
热度:69
Tijmen Tieleman(多伦多大学) A new algorithm for training Restricted Boltzmann Machines is introduced. The algorithm, named Persistent Contrastive Divergence, is different from th...
热度:69

Nonparametric Bayesian Density Modeling with Gaussian Processes[高斯过程的非参数贝叶斯密度建模]
Ryan Prescott Adams(多伦多大学) We present the Gaussian Process Density Sampler (GPDS), an exchangeable generative model for use in nonparametric Bayesian density estimation. Sample...
热度:111
Ryan Prescott Adams(多伦多大学) We present the Gaussian Process Density Sampler (GPDS), an exchangeable generative model for use in nonparametric Bayesian density estimation. Sample...
热度:111
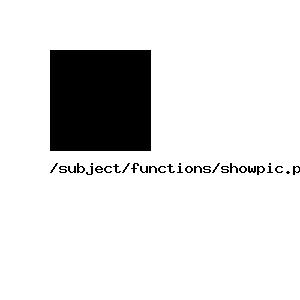
Three New Graphical Models for Statistical Language Modelling[统计语言建模的三种新图形模型]
Andriy Mnih(多伦多大学) The supremacy of n-gram models in statistical language modelling has recently been challenged by parametric models that use distributed representation...
热度:117
Andriy Mnih(多伦多大学) The supremacy of n-gram models in statistical language modelling has recently been challenged by parametric models that use distributed representation...
热度:117

Kernel-Based Copula Processes[基于内核的Copula流程]
Eddie K. H. Ng(多伦多大学) Kernel-based Copula Processes (KCPs), a new versatile tool for analyzing multiple time-series, are proposed here as a unifying framework to model the ...
热度:95
Eddie K. H. Ng(多伦多大学) Kernel-based Copula Processes (KCPs), a new versatile tool for analyzing multiple time-series, are proposed here as a unifying framework to model the ...
热度:95