开课单位--卡内基梅隆大学
101
102
103
104
105
106
107
108
109
110
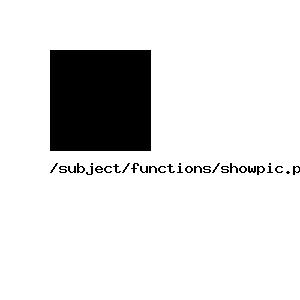
Adaptive Representations for Efficient Inference for Distributions on Permutations[排列分布有效推理的自适应表示]
Carlos Guestrin(卡内基梅隆大学) Permutations are ubiquitous in many real world problems, such as voting, rankings and data association. Representing uncertainty over permutations is ...
热度:35
Carlos Guestrin(卡内基梅隆大学) Permutations are ubiquitous in many real world problems, such as voting, rankings and data association. Representing uncertainty over permutations is ...
热度:35
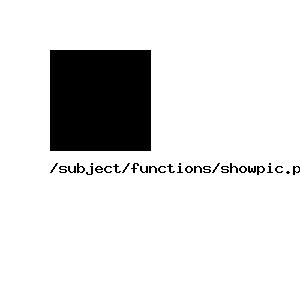
A theory of similarity functions for learning and clustering[学习和聚类的相似函数理论]
Avrim Blum(卡内基梅隆大学) Kernel methods have proven to be very powerful tools in machine learning. In addition, there is a well-developed theory of sufficient conditions for a...
热度:47
Avrim Blum(卡内基梅隆大学) Kernel methods have proven to be very powerful tools in machine learning. In addition, there is a well-developed theory of sufficient conditions for a...
热度:47

Undercover: Authentication Usable in Front of Prying Eyes[卧底:在窥探前可用的认证]
Eiji Hayashi(卡内基梅隆大学) We propose the first authentication scheme to rely on the humanability to combine different sensory inputs. We demonstrate thesystem is usable and res...
热度:26
Eiji Hayashi(卡内基梅隆大学) We propose the first authentication scheme to rely on the humanability to combine different sensory inputs. We demonstrate thesystem is usable and res...
热度:26


A Unified View of Matrix Factorization Models[矩阵分解模型的统一视图]
Ajit Singh;Geoffrey J. Gordon(卡内基梅隆大学) We present a unified view of matrix factorization that frames the differences among popular methods, such as NMF, Weighted SVD, E-PCA, MMMF, pLSI, pLS...
热度:33
Ajit Singh;Geoffrey J. Gordon(卡内基梅隆大学) We present a unified view of matrix factorization that frames the differences among popular methods, such as NMF, Weighted SVD, E-PCA, MMMF, pLSI, pLS...
热度:33

Designing Online Communities from Theory[用理论设计网络社区]
Bob Kraut(卡内基梅隆大学) Online communities are the fastest-growing portion of the Internet and provide members with information, social support, and entertainment. While a mi...
热度:35
Bob Kraut(卡内基梅隆大学) Online communities are the fastest-growing portion of the Internet and provide members with information, social support, and entertainment. While a mi...
热度:35
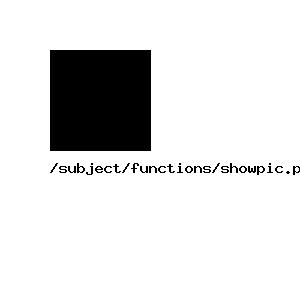
Rare Category Detection for Spatial Data[空间数据的罕见类别检测]
Jingrui He(卡内基梅隆大学) Given an unlabeled unbalanced data set, the goal of rare category detection is to discover examples from the minority classes with a few label request...
热度:42
Jingrui He(卡内基梅隆大学) Given an unlabeled unbalanced data set, the goal of rare category detection is to discover examples from the minority classes with a few label request...
热度:42

Relational Learning as Collective Matrix Factorization[集体矩阵分解的关系学习]
Ajit Singh(卡内基梅隆大学) We present a unified view of matrix factorization models, including singular value decompositions, non-negative matrix factorization, probabilistic la...
热度:78
Ajit Singh(卡内基梅隆大学) We present a unified view of matrix factorization models, including singular value decompositions, non-negative matrix factorization, probabilistic la...
热度:78
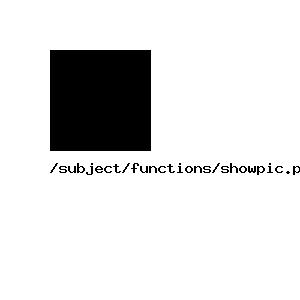
Partially Observed Maximum Entropy Discrimination Markov Networks[部分可观测的最大熵辨别马尔可夫网络]
Jun Zhu(卡内基梅隆大学) Learning graphical models with hidden variables can offer semantic insights to complex data and lead to salient structured predictors without relying ...
热度:39
Jun Zhu(卡内基梅隆大学) Learning graphical models with hidden variables can offer semantic insights to complex data and lead to salient structured predictors without relying ...
热度:39

Discovering Cyclic Causal Models by Independent Components Analysis[通过独立成分分析发现循环因果模型]
Gustavo Lacerda(卡内基梅隆大学) This talk will start by presenting Shimizu et al's (2006) ICA-based approach (LiNGAM) for discovering acyclic (DAG) linear Structural Equation Mod...
热度:50
Gustavo Lacerda(卡内基梅隆大学) This talk will start by presenting Shimizu et al's (2006) ICA-based approach (LiNGAM) for discovering acyclic (DAG) linear Structural Equation Mod...
热度:50