开课单位--卡内基梅隆大学
51
52
53
54
55
56
57
58
59
60
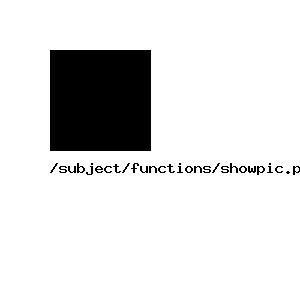
Pose Machines: Articulated Pose Estimation via Inference Machines[姿态机:通过推理机进行关节姿态估计]
Varun Ramakrishna(卡内基梅隆大学) State-of-the-art approaches for articulated human pose estimation are rooted in parts-based graphical models. These models are often restricted to tre...
热度:42
Varun Ramakrishna(卡内基梅隆大学) State-of-the-art approaches for articulated human pose estimation are rooted in parts-based graphical models. These models are often restricted to tre...
热度:42
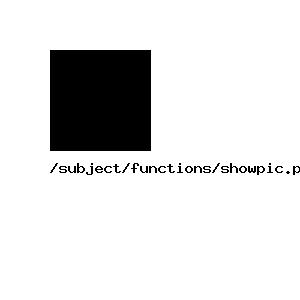
Mining Billion-node Graphs: Patterns, Generators and Tools[挖掘亿节点图:模式,发电机和工具]
Christos Faloutsos(卡内基梅隆大学) What do graphs look like? How do they evolve over time? How to handle a graph with a billion nodes? We present a comprehensive list of static and temp...
热度:62
Christos Faloutsos(卡内基梅隆大学) What do graphs look like? How do they evolve over time? How to handle a graph with a billion nodes? We present a comprehensive list of static and temp...
热度:62
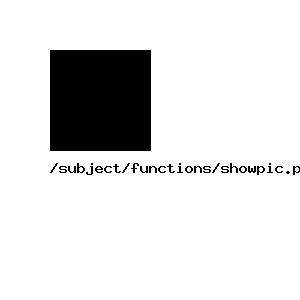
REGO: Rank-based estimation of Renyi information using Euclidean graph optimization[REGO:基于欧氏图优化的Renyi信息秩估计]
Barnabás Póczos(卡内基梅隆大学) We propose a new method for a non-parametric estimation of Renyi and Shannon information for a multivariate distribution using a corresponding copula,...
热度:32
Barnabás Póczos(卡内基梅隆大学) We propose a new method for a non-parametric estimation of Renyi and Shannon information for a multivariate distribution using a corresponding copula,...
热度:32

Interview with Tom Mitchell[汤姆米切尔访谈]
Tom Mitchell, Davor Orlič(卡内基梅隆大学) **Tom Mitchell is the first Chair of Department of the first Machine Learning Department in the World**, based at Carnegie Mellon. The Videolectures.N...
热度:52
Tom Mitchell, Davor Orlič(卡内基梅隆大学) **Tom Mitchell is the first Chair of Department of the first Machine Learning Department in the World**, based at Carnegie Mellon. The Videolectures.N...
热度:52
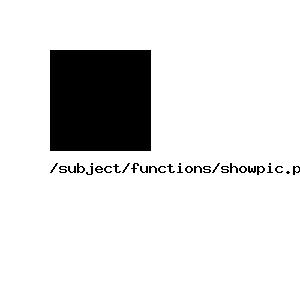
Domain Adaptation for Mobile Robot Navigation[移动机器人导航域适配 ]
David Bradley(卡内基梅隆大学) An important challenge in outdoor mobile robotic perception is maintaining terrain classification performance throughout the extremely variable condit...
热度:67
David Bradley(卡内基梅隆大学) An important challenge in outdoor mobile robotic perception is maintaining terrain classification performance throughout the extremely variable condit...
热度:67
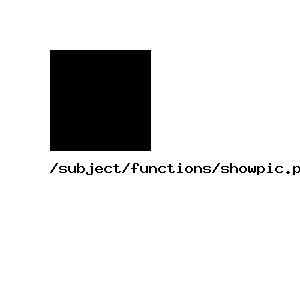
Data-Driven Scene Understanding from 3D Models[3D模型中的数据驱动场景理解]
Scott Satkin(卡内基梅隆大学) In this paper, we propose a data-driven approach to leverage repositories of 3D models for scene understanding. Our ability to relate what we see in a...
热度:139
Scott Satkin(卡内基梅隆大学) In this paper, we propose a data-driven approach to leverage repositories of 3D models for scene understanding. Our ability to relate what we see in a...
热度:139
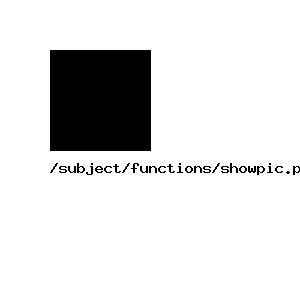
The Web Changes Everything: Understanding the Dynamics of Web Content[互联网改变了一切:了解网页内容的动态性]
Susan T. Dumais;Jaime Teevan;Jonathan Elsas;Eytan Adar(卡内基梅隆大学) The Web Changes Everything: Understanding the Dynamics of Web Content
热度:26
Susan T. Dumais;Jaime Teevan;Jonathan Elsas;Eytan Adar(卡内基梅隆大学) The Web Changes Everything: Understanding the Dynamics of Web Content
热度:26

Fast learning of Document Ranking Functions with the Committee Perceptron[快速学习委员会感知器的文档排序功能]
Jonathan Elsas(卡内基梅隆大学) Fast learning of Document Ranking Functions with the Committee Perceptron
热度:28
Jonathan Elsas(卡内基梅隆大学) Fast learning of Document Ranking Functions with the Committee Perceptron
热度:28

Semi-Supervised Learning and Learning via Similarity Functions: two key settings for Data-dependent Concept Spaces[基于相似函数的半监督学习:数据相关概念空间的两种关键设置]
Avrim Blum(卡内基梅隆大学) Semi-Supervised Learning and Learning via Similarity Functions: two key settings for Data-dependent Concept Spaces
热度:77
Avrim Blum(卡内基梅隆大学) Semi-Supervised Learning and Learning via Similarity Functions: two key settings for Data-dependent Concept Spaces
热度:77
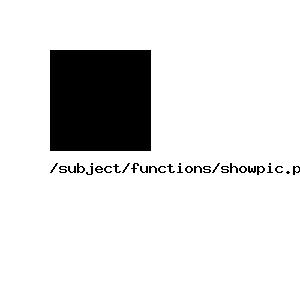
A Reduction of Imitation Learning and Structured Prediction to No-Regret Online Learning[减少模仿学习和结构化预测的没遗憾的在线学习]
Stephane Ross(卡内基梅隆大学) Sequential prediction problems such as imitation learning, where future observations depend on previous predictions (actions), violate the common i.i....
热度:82
Stephane Ross(卡内基梅隆大学) Sequential prediction problems such as imitation learning, where future observations depend on previous predictions (actions), violate the common i.i....
热度:82