开课单位--卡内基梅隆大学
41
42
43
44
45
46
47
48
49
50
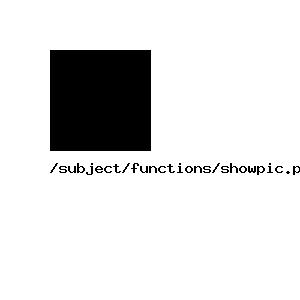
Reducing the Sampling Complexity of Topic Models[降低主题模型的抽样复杂度]
Aaron Li(卡内基梅隆大学) Inference in topic models typically involves a sampling step to associate latent variables with observations. Unfortunately the generative model loses...
热度:34
Aaron Li(卡内基梅隆大学) Inference in topic models typically involves a sampling step to associate latent variables with observations. Unfortunately the generative model loses...
热度:34

Opinion Fraud Detection in Online Reviews by Network Effects[基于网络效应的在线评论意见欺诈检测]
Leman Akoglu(卡内基梅隆大学) User-generated online reviews can play a significant role in the success of retail products, hotels, restaurants, etc. However,review systems are ofte...
热度:70
Leman Akoglu(卡内基梅隆大学) User-generated online reviews can play a significant role in the success of retail products, hotels, restaurants, etc. However,review systems are ofte...
热度:70
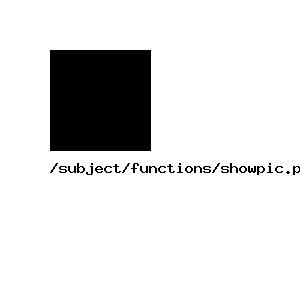
A Globally Optimal Data-Driven Approach for Image Distortion Estimation[一种全局最优数据驱动的图像失真估计方法]
Yuandong Tian(卡内基梅隆大学) Image alignment in the presence of non-rigid distortions is a challenging task. Typically, this involves estimating the parameters of a dense deformat...
热度:34
Yuandong Tian(卡内基梅隆大学) Image alignment in the presence of non-rigid distortions is a challenging task. Typically, this involves estimating the parameters of a dense deformat...
热度:34
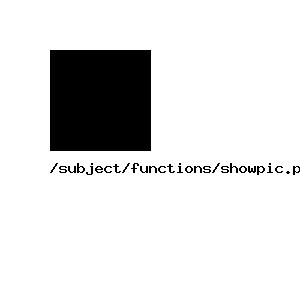
Data-Driven Scene Understanding from 3D Models[基于三维模型的数据驱动场景理解]
Scott Satkin(卡内基梅隆大学) In this paper, we propose a data-driven approach to leverage repositories of 3D models for scene understanding. Our ability to relate what we see in a...
热度:58
Scott Satkin(卡内基梅隆大学) In this paper, we propose a data-driven approach to leverage repositories of 3D models for scene understanding. Our ability to relate what we see in a...
热度:58

How Optimized Environmental Sensing Helps Address Information Overload on the Web[优化的环境感知如何帮助解决网络上的信息过载问题]
Tom Mitchell(卡内基梅隆大学) In this talk, we tackle a fundamental problem that arises when using sensors to monitor the ecological condition of rivers and lakes, the network of p...
热度:3
Tom Mitchell(卡内基梅隆大学) In this talk, we tackle a fundamental problem that arises when using sensors to monitor the ecological condition of rivers and lakes, the network of p...
热度:3
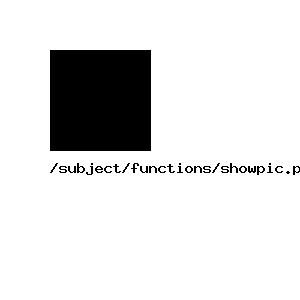
The sample complexity of agnostic learning under deterministic labels[确定性标签下不可知学习的样本复杂度]
Ruth Urner(卡内基梅隆大学) With the emergence of Machine Learning tools that allow handling data with a huge number of features, it becomes reasonable to assume that, over the f...
热度:38
Ruth Urner(卡内基梅隆大学) With the emergence of Machine Learning tools that allow handling data with a huge number of features, it becomes reasonable to assume that, over the f...
热度:38
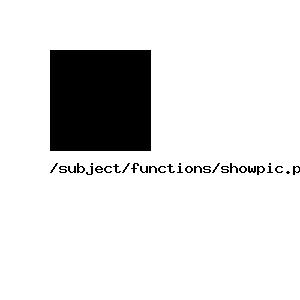
Jointly Modeling Aspects, Ratings and Sentiments for Movie Recommendation (JMARS)[联合建模方面,收视率和情感电影推荐(JMARS)]
Chao-Yuan Wu(卡内基梅隆大学) Recommendation and review sites offer a wealth of information beyond ratings. For instance, on IMDb users leave reviews, commenting on different aspec...
热度:85
Chao-Yuan Wu(卡内基梅隆大学) Recommendation and review sites offer a wealth of information beyond ratings. For instance, on IMDb users leave reviews, commenting on different aspec...
热度:85
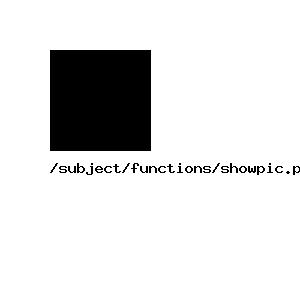
Should Model Architecture Reflect Linguistic Structure?[模型建筑应该反映语言结构吗?]
Chris Dyer(卡内基梅隆大学) Sequential recurrent neural networks (RNNs) over finite alphabets are remarkably effective models of natural language. RNNs now obtain language modeli...
热度:22
Chris Dyer(卡内基梅隆大学) Sequential recurrent neural networks (RNNs) over finite alphabets are remarkably effective models of natural language. RNNs now obtain language modeli...
热度:22
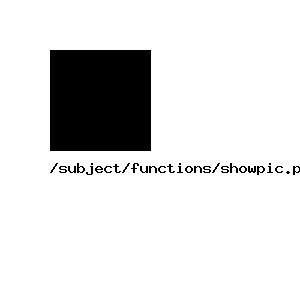
Learning Deep Generative Models[学习深层生成模型]
Ruslan Salakhutdinov(卡内基梅隆大学) In this tutorial I will discuss mathematical basics of many popular deep generative models, including Restricted Boltzmann Machines (RBMs), Deep Boltz...
热度:29
Ruslan Salakhutdinov(卡内基梅隆大学) In this tutorial I will discuss mathematical basics of many popular deep generative models, including Restricted Boltzmann Machines (RBMs), Deep Boltz...
热度:29
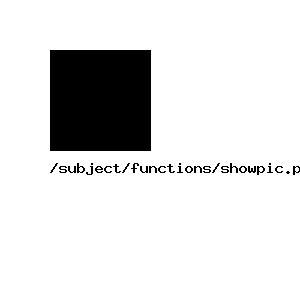
Unfolding an Indoor Origami World[展开室内折纸世界]
David Ford Fouhey(卡内基梅隆大学) In this work, we present a method for single-view reasoning about 3D surfaces and their relationships. We propose the use of mid-level constraints for...
热度:33
David Ford Fouhey(卡内基梅隆大学) In this work, we present a method for single-view reasoning about 3D surfaces and their relationships. We propose the use of mid-level constraints for...
热度:33