开课单位--东京大学
11
12
13
14
15
16
17
18
19
20
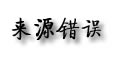
Controlling Humanoid Robots by Means of Genetic Programming[基于遗传规划的仿人机器人控制]
Hitoshi Iba(东京大学) We show the real-world applications of EC (evolutionary computation) to robotics, which is called "evolutionary robotics". Machine Learning ...
热度:37
Hitoshi Iba(东京大学) We show the real-world applications of EC (evolutionary computation) to robotics, which is called "evolutionary robotics". Machine Learning ...
热度:37
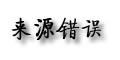
Measuring the Similarity between Implicit Semantic Relations from the Web[从Web中测量隐含语义关系的相似性]
Danushka Bollegala;Yutaka Matsuo;Mitsuru Ishizuka(东京大学) Measuring the similarity between semantic relations that hold among entities is an important and necessary step in various Web related tasks such as r...
热度:60
Danushka Bollegala;Yutaka Matsuo;Mitsuru Ishizuka(东京大学) Measuring the similarity between semantic relations that hold among entities is an important and necessary step in various Web related tasks such as r...
热度:60
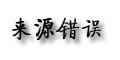
Baryon Asymmetry of the Universe[宇宙的重子不对称性]
Hitoshi Murayama(东京大学) In two lectures, the following topics will be discussed: (1) Why baryon asymmetry is a problem at all (2) Review of the Sakharov's conditions (3) Why...
热度:48
Hitoshi Murayama(东京大学) In two lectures, the following topics will be discussed: (1) Why baryon asymmetry is a problem at all (2) Review of the Sakharov's conditions (3) Why...
热度:48
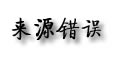
Eigenmode of Decision-by-Majority Process on Complex Networks[复杂网络上多数决策过程的特征模式]
Makoto Uchida(东京大学) The nature of opinion formation dynamics in complex networks is investigated using eigenmode analysis. Opinion formation dynamics is modeled by a deci...
热度:105
Makoto Uchida(东京大学) The nature of opinion formation dynamics in complex networks is investigated using eigenmode analysis. Opinion formation dynamics is modeled by a deci...
热度:105
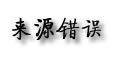
Collusion-Resistant Privacy-Preserving Data Mining[防止串通的隐私保护数据挖掘]
Bin Yang(东京大学) Recent research in privacy-preserving data mining (PPDM) has become increasingly popular due to the wide application of data mining and the increased ...
热度:61
Bin Yang(东京大学) Recent research in privacy-preserving data mining (PPDM) has become increasingly popular due to the wide application of data mining and the increased ...
热度:61
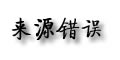
Knowledge Discovery of Multiple-topic Document using Parametric Mixture Model with Dirichlet Prior[基于Dirichlet先验参数混合模型的多主题文献知识发现]
Issei Sato(东京大学) Documents, such as those seen onWikipedia and Folksonomy, have tended to be assigned with multiple topics as a meta-data. Therefore, it is more and mo...
热度:35
Issei Sato(东京大学) Documents, such as those seen onWikipedia and Folksonomy, have tended to be assigned with multiple topics as a meta-data. Therefore, it is more and mo...
热度:35
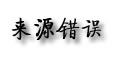
Proto-Predictive Representation of States with Simple Recurrent Temporal-Difference Networks[具有简单递归时差网络的状态的原始预测表示]
Takaki Makino(东京大学) We propose a new neural network architecture, called Simple Recurrent Temporal-Difference Networks (SR-TDNs), that learns to predict future observatio...
热度:37
Takaki Makino(东京大学) We propose a new neural network architecture, called Simple Recurrent Temporal-Difference Networks (SR-TDNs), that learns to predict future observatio...
热度:37
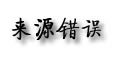
On-line Discovery of Temporal-Difference Networks[时间差网络的在线发现]
Takaki Makino(东京大学) We present an algorithm for on-line, incremental discovery of temporal-difference (TD) networks. The key contribution is the establishment of three cr...
热度:55
Takaki Makino(东京大学) We present an algorithm for on-line, incremental discovery of temporal-difference (TD) networks. The key contribution is the establishment of three cr...
热度:55
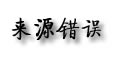
Frequency-aware Truncated methods for Sparse Online Learning[用于稀疏在线学习的频率感知截断方法]
Hidekazu Oiwa(东京大学) Online supervised learning with L1-regularization has gained attention recently because it generally requires less computational time and a smaller sp...
热度:65
Hidekazu Oiwa(东京大学) Online supervised learning with L1-regularization has gained attention recently because it generally requires less computational time and a smaller sp...
热度:65
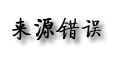
Mining Peculiar Compositions of Frequent Substrings from Sparse Text Data Using Background Texts[利用背景文本从稀疏文本数据中挖掘频繁字符串的特殊成分]
Daisuke Ikeda(东京大学) We consider mining unusual patterns from text T. Unlike existing methods which assume probabilistic models and use simple estimation methods, we emplo...
热度:37
Daisuke Ikeda(东京大学) We consider mining unusual patterns from text T. Unlike existing methods which assume probabilistic models and use simple estimation methods, we emplo...
热度:37