开课单位--加州大学伯克利分校
51
52
53
54
55
56
57
58
59
60
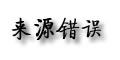
Seeking Interpretable Models for High Dimensional Data[寻找高维数据的可解释模型]
Bin Yu(加州大学伯克利分校) Extracting useful information from high-dimensional data is the focus of today's statistical research and practice. After broad success of statistical...
热度:76
Bin Yu(加州大学伯克利分校) Extracting useful information from high-dimensional data is the focus of today's statistical research and practice. After broad success of statistical...
热度:76
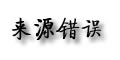
AdaBoost is Universally Consistent[演算法是普遍一致的]
Peter L. Bartlett(加州大学伯克利分校) We consider the risk, or probability of error, of the classifier produced by AdaBoost, and in particular the stopping strategy to be used to ensure un...
热度:41
Peter L. Bartlett(加州大学伯克利分校) We consider the risk, or probability of error, of the classifier produced by AdaBoost, and in particular the stopping strategy to be used to ensure un...
热度:41
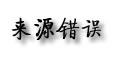
Inference for Networks[对网络的推断]
Peter J. Bickel(加州大学伯克利分校) A great deal of attention has recently been paid to determining sub-communities on the basis of relations, corresponding to edges, between individuals...
热度:36
Peter J. Bickel(加州大学伯克利分校) A great deal of attention has recently been paid to determining sub-communities on the basis of relations, corresponding to edges, between individuals...
热度:36
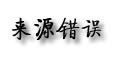
Pattern Classification and Large Margin Classifiers[模式分类和大边距分类器]
Peter L. Bartlett(加州大学伯克利分校) These lectures will provide an introduction to the theory of pattern classification methods. They will focus on relationships between the minimax perf...
热度:75
Peter L. Bartlett(加州大学伯克利分校) These lectures will provide an introduction to the theory of pattern classification methods. They will focus on relationships between the minimax perf...
热度:75
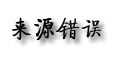
On the Borders of Statistics and Computer Science[论统计与计算机科学的边界]
Peter J. Bickel(加州大学伯克利分校) Machine learning in computer science and prediction and classification in statistics are essentially equivalent fields. I will try to illustrate the r...
热度:62
Peter J. Bickel(加州大学伯克利分校) Machine learning in computer science and prediction and classification in statistics are essentially equivalent fields. I will try to illustrate the r...
热度:62
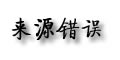
On the Adequacy of Baseform Pronunciations and Pronunciation Variants[论基线发音和发音变体的充分性]
Chuck Wooters(加州大学伯克利分校) This paper presents an approach to automatically extract and evaluate the ``stability'' of pronunciation variants (i.e., adequacy of the model to acco...
热度:62
Chuck Wooters(加州大学伯克利分校) This paper presents an approach to automatically extract and evaluate the ``stability'' of pronunciation variants (i.e., adequacy of the model to acco...
热度:62
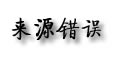
Horizon-Independent Optimal Prediction with Log-Loss in Exponential Families[指数族中具有对数损失水平的独立最优预测]
Fares Hedayati(加州大学伯克利分校) We study online learning under logarithmic loss with regular parametric models. Hedayati and Bartlett (2012) showed that a Bayesian prediction strateg...
热度:40
Fares Hedayati(加州大学伯克利分校) We study online learning under logarithmic loss with regular parametric models. Hedayati and Bartlett (2012) showed that a Bayesian prediction strateg...
热度:40
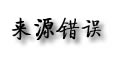
Machine Learning for Robotics[机器人的学习能力]
Pieter Abbeel(加州大学伯克利分校) Robots are typically far less capable in autonomous mode than in tele-operated mode. The few exceptions tend to stem from long days (and more often we...
热度:119
Pieter Abbeel(加州大学伯克利分校) Robots are typically far less capable in autonomous mode than in tele-operated mode. The few exceptions tend to stem from long days (and more often we...
热度:119
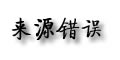
Near Optimal Chernoff Bounds for Markov Decision Processes[马尔可夫决策过程的近最佳Chernoff界]
Teodor Mihai Moldovan(加州大学伯克利分校) The expected return is a widely used objective in decision making under uncertainty. Many algorithms, such as value iteration, have been proposed to o...
热度:57
Teodor Mihai Moldovan(加州大学伯克利分校) The expected return is a widely used objective in decision making under uncertainty. Many algorithms, such as value iteration, have been proposed to o...
热度:57
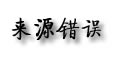
Group Lasso with Overlaps and Graph Lasso[使用Overlaps和Graph Lasso组合套索]
Laurent Jacob(加州大学伯克利分校) We propose a new penalty function which, when used as regularization for empirical risk minimization procedures, leads to sparse estimators. The suppo...
热度:89
Laurent Jacob(加州大学伯克利分校) We propose a new penalty function which, when used as regularization for empirical risk minimization procedures, leads to sparse estimators. The suppo...
热度:89