开课单位--斯坦福大学
191
192
193
194
195
196
197
198
199
200
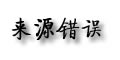
CourseRank: A Closed-Community Social System through the Magnifying Glass[课程等级:放大镜下的封闭社区社会系统 ]
Koutrika Georgia(斯坦福大学) Social sites are extremely popular among users but user interactions in most sites revolve around relatively simple tasks, such as uploading resources...
热度:63
Koutrika Georgia(斯坦福大学) Social sites are extremely popular among users but user interactions in most sites revolve around relatively simple tasks, such as uploading resources...
热度:63
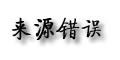
Large-Scale Deep Unsupervised Learning Using Graphics Processors[使用图形处理器进行大规模深度无监督学习]
Rajat Raina(斯坦福大学) The promise of unsupervised learning methods lies in their potential to use vast amounts of unlabeled data to learn complex, highly nonlinear models w...
热度:26
Rajat Raina(斯坦福大学) The promise of unsupervised learning methods lies in their potential to use vast amounts of unlabeled data to learn complex, highly nonlinear models w...
热度:26
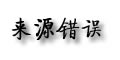
Unsupervised Discovery of Structure, Succinct Representations and Sparsity[结构的无监督发现、简洁的表示和稀疏性]
Andrew Ng(斯坦福大学) We describe a class of unsupervised learning methods that learn sparse representations of the training data, and thereby identify useful features. Fur...
热度:38
Andrew Ng(斯坦福大学) We describe a class of unsupervised learning methods that learn sparse representations of the training data, and thereby identify useful features. Fur...
热度:38
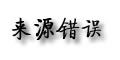
Reinforcement Learning, Apprenticeship Learning and Robotic Control[强化学习,学徒学习和机器人控制]
Andrew Ng(斯坦福大学) Reinforcement learning has proved to be a powerful method for robotic control. In this talk, drawing on examples from autonomous helicopter flight, qu...
热度:55
Andrew Ng(斯坦福大学) Reinforcement learning has proved to be a powerful method for robotic control. In this talk, drawing on examples from autonomous helicopter flight, qu...
热度:55
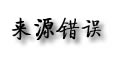
Statistical Leverage and Improved Matrix Algorithms[统计杠杆和改进的矩阵算法]
Michael Mahoney(斯坦福大学) Given an m x n matrix A and a rank parameter k, define the leverage of the i-th row of A to be the i-th diagonal element of the projection matrix onto...
热度:89
Michael Mahoney(斯坦福大学) Given an m x n matrix A and a rank parameter k, define the leverage of the i-th row of A to be the i-th diagonal element of the projection matrix onto...
热度:89
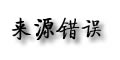
Modeling Social and Information Networks: Opportunities for Machine Learning[社交和信息网络建模:机器学习的机会]
Jure Leskovec(斯坦福大学) Emergence of the web, social media and online social networking websites gave rise to detailed traces of human social activity. This offers many oppor...
热度:31
Jure Leskovec(斯坦福大学) Emergence of the web, social media and online social networking websites gave rise to detailed traces of human social activity. This offers many oppor...
热度:31
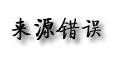
STAIR: The STanford Artificial Intelligence Robot project[STAIR:STanford人工智能机器人项目]
Andrew Ng, Andrew McCallum(斯坦福大学) This talk will describe the STAIR home assistant robot project, and several satellite projects that led to key STAIR components such as (i) robotic gr...
热度:84
Andrew Ng, Andrew McCallum(斯坦福大学) This talk will describe the STAIR home assistant robot project, and several satellite projects that led to key STAIR components such as (i) robotic gr...
热度:84
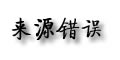
Fast Gaussian Process Methods for Point Process Intensity Estimation[点过程强度估计的快速高斯处理方法]
John Cunningham(斯坦福大学) Point processes are difficult to analyze because they provide only a sparse and noisy observation of the intensity function driving the process. Gauss...
热度:64
John Cunningham(斯坦福大学) Point processes are difficult to analyze because they provide only a sparse and noisy observation of the intensity function driving the process. Gauss...
热度:64
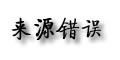
Scalable Modeling of Real Graphs using Kronecker Multiplication[基于Kronecker乘法的实图可扩展建模]
Jure Leskovec(斯坦福大学) Given a large, real graph, how can we generate a synthetic graph that matches its properties, i.e., it has similar degree distribution, similar (small...
热度:57
Jure Leskovec(斯坦福大学) Given a large, real graph, how can we generate a synthetic graph that matches its properties, i.e., it has similar degree distribution, similar (small...
热度:57
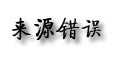
Classifying single trial fMRI: What can machine learning learn?[单次试验功能磁共振的分类:机器学习能学到什么?]
Paul Mazaika(斯坦福大学) We describe three experiments combining neuroimaging and machine learning. The first experiment compares the performance of maximum likelihood and ne...
热度:88
Paul Mazaika(斯坦福大学) We describe three experiments combining neuroimaging and machine learning. The first experiment compares the performance of maximum likelihood and ne...
热度:88