开课单位--加州大学
1
2
3
4
5
6
7
8
9
10
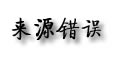
Matrix Profile V: A Generic Technique to Incorporate Domain Knowledge into Motif Discovery[矩阵配置文件 V:将领域知识纳入主题发现的通用技术]
Hoang Anh Dau(加州大学) Time series motif discovery has emerged as perhaps the most used primitive for time series data mining, and has seen applications to domains as divers...
热度:29
Hoang Anh Dau(加州大学) Time series motif discovery has emerged as perhaps the most used primitive for time series data mining, and has seen applications to domains as divers...
热度:29
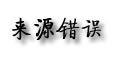
Convex Risk Minimization and Conditional Probability Estimation[凸风险最小化与条件概率估计]
Matus Telgarsky(加州大学) This manuscript strengthens the link between convex risk minimization and conditional probability estimation, a connection already notable for establi...
热度:43
Matus Telgarsky(加州大学) This manuscript strengthens the link between convex risk minimization and conditional probability estimation, a connection already notable for establi...
热度:43
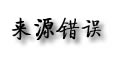
Detecting the Presence and Absence of Causal Relationships Between Expression of Yeast Genes with Very Few Samples[用很少的样品检测酵母基因表达之间因果关系的存在与否]
Eun Yong Kang(加州大学) Detecting the Presence and Absence of Causal Relationships Between Expression of Yeast Genes with Very Few Samples
热度:36
Eun Yong Kang(加州大学) Detecting the Presence and Absence of Causal Relationships Between Expression of Yeast Genes with Very Few Samples
热度:36
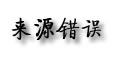
Kernel Choice and Classifiability for RKHS Embeddings of Probability Distributions[概率分布RKHS嵌入的核选择和可分类性]
Bharath K.Sriperumbudur(加州大学) Embeddings of probability measures into reproducing kernel Hilbert spaces have been proposed as a straightforward and practical means of representing ...
热度:73
Bharath K.Sriperumbudur(加州大学) Embeddings of probability measures into reproducing kernel Hilbert spaces have been proposed as a straightforward and practical means of representing ...
热度:73
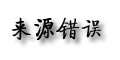
Boosting with Structural Sparsity[结构稀疏的增压]
John Duchi(加州大学) We derive generalizations of AdaBoost and related gradient-based coordinate descent methods that incorporate sparsity-promoting penalties for the norm...
热度:47
John Duchi(加州大学) We derive generalizations of AdaBoost and related gradient-based coordinate descent methods that incorporate sparsity-promoting penalties for the norm...
热度:47
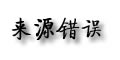
Accounting for Burstiness in Topic Models[主题模型中突发事件的解释]
Gabriel Doyle(加州大学) Many different topic models have been used successfully for a variety of applications. However, even state-of-the-art topic models suffer from the imp...
热度:35
Gabriel Doyle(加州大学) Many different topic models have been used successfully for a variety of applications. However, even state-of-the-art topic models suffer from the imp...
热度:35
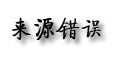
Lower bounds on the performance of polynomial-time algorithms for sparse linear regression[稀疏线性回归多项式时间算法性能的下界]
Yuchen Zhang(加州大学) Under a standard assumption in complexity theory (NP not in P/poly), we demonstrate a gap between the minimax prediction risk for sparse linear regres...
热度:41
Yuchen Zhang(加州大学) Under a standard assumption in complexity theory (NP not in P/poly), we demonstrate a gap between the minimax prediction risk for sparse linear regres...
热度:41
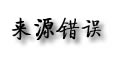
Large-Scale Markov Decision Problems with KL Control Cost and its Application to Crowdsourcing[具有KL控制成本的大规模Markov决策问题及其在众包中的应用]
Alan Malek(加州大学) We study average and total cost Markov decision problems with large state spaces. Since the computational and statistical costs of finding the optimal...
热度:43
Alan Malek(加州大学) We study average and total cost Markov decision problems with large state spaces. Since the computational and statistical costs of finding the optimal...
热度:43
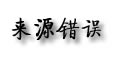
Causal Inference as Computational Learning[作为计算学习的因果推理]
Judea Pearl,(加州大学) Causal Inference as Computational Learning
热度:32
Judea Pearl,(加州大学) Causal Inference as Computational Learning
热度:32
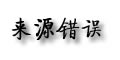