开课单位--马克斯普朗克研究所
91
92
93
94
95
96
97
98
99
100
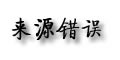
Weighted Substructure Mining for Image Analysis[图像分析的加权子结构挖掘]
Sebastian Nowozin(马克斯普朗克研究所) In web-related applications of image categorization, it is desirable to derive an interpretable classification rule with high accuracy. Using the ...
热度:14
Sebastian Nowozin(马克斯普朗克研究所) In web-related applications of image categorization, it is desirable to derive an interpretable classification rule with high accuracy. Using the ...
热度:14
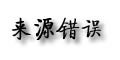
Psychoacoustic Influences on the Neural Correlates of music Syntactic Processing[心理声学对音乐句法加工神经相关性的影响]
Sebastian Jentschke(马克斯普朗克研究所) Music consists of perceptually discrete elements that are organized according to syntactic regularities. Violations of these regularities typically el...
热度:55
Sebastian Jentschke(马克斯普朗克研究所) Music consists of perceptually discrete elements that are organized according to syntactic regularities. Violations of these regularities typically el...
热度:55
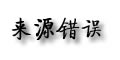
Semantic Knowledge Bases from Web Sources[基于Web源的语义知识库]
Hady W. Lauw, Fabian M. Suchanek, Ralf Schenkel, Martin Theobald, Gerhard Weikum(马克斯普朗克研究所) The advent of knowledge-sharing communities such as Wikipedia and the progress in scalable information extraction from Web sources has enabled the aut...
热度:26
Hady W. Lauw, Fabian M. Suchanek, Ralf Schenkel, Martin Theobald, Gerhard Weikum(马克斯普朗克研究所) The advent of knowledge-sharing communities such as Wikipedia and the progress in scalable information extraction from Web sources has enabled the aut...
热度:26
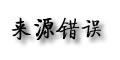
Measuring User Influence on Twitter: The Million Follower Fallacy[衡量用户对Twitter的影响:百万追随者谬论]
Meeyoung Cha(马克斯普朗克研究所) Directed links in social media could represent anything from intimate friendships to common interests, or even a passion for breaking news or celebrit...
热度:36
Meeyoung Cha(马克斯普朗克研究所) Directed links in social media could represent anything from intimate friendships to common interests, or even a passion for breaking news or celebrit...
热度:36
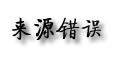
A note of caution regarding distances on graphs[关于图上距离的注意事项]
Ulrike von Luxburg(马克斯普朗克研究所) Non-geometric data is often represented in form of a graph where edges represent similarity or local relationships between instances. One elegant way ...
热度:26
Ulrike von Luxburg(马克斯普朗克研究所) Non-geometric data is often represented in form of a graph where edges represent similarity or local relationships between instances. One elegant way ...
热度:26
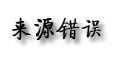
Solution Stability in Linear Programming Relaxations: Graph Partitioning and Unsupervised Learning[线性规划松弛的解稳定性:图分割和无监督学习]
Sebastian Nowozin(马克斯普朗克研究所) We propose a new method to quantify the solution stability of a large class of combinatorial optimization problems arising in machine learning. As pra...
热度:83
Sebastian Nowozin(马克斯普朗克研究所) We propose a new method to quantify the solution stability of a large class of combinatorial optimization problems arising in machine learning. As pra...
热度:83
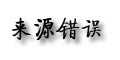
Convex Variational Bayesian Inference for Large Scale Generalized Linear Models[大尺度广义线性模型的凸变分贝叶斯推理]
Hannes Nickisch(马克斯普朗克研究所) We show how variational Bayesian inference can be implemented for very large generalized linear models. Our relaxation is proven to be a convex proble...
热度:21
Hannes Nickisch(马克斯普朗克研究所) We show how variational Bayesian inference can be implemented for very large generalized linear models. Our relaxation is proven to be a convex proble...
热度:21
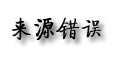
Sparse Multiscale Gaussian Process Regression[稀疏多尺度高斯过程回归]
Christian Walder(马克斯普朗克研究所) Most existing sparse Gaussian process (g.p.) models seek computational advantages by basing their computations on a set of m basis functions that are ...
热度:192
Christian Walder(马克斯普朗克研究所) Most existing sparse Gaussian process (g.p.) models seek computational advantages by basing their computations on a set of m basis functions that are ...
热度:192
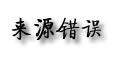
Gaussian Process Product Models for Nonparametric Nonstationarity[非参数非平稳性的高斯过程产品模型]
Oliver Stegle(马克斯普朗克研究所) Stationarity is often an unrealistic prior assumption for Gaussian process regression. One solution is to predefine an explicit nonstationary covarian...
热度:69
Oliver Stegle(马克斯普朗克研究所) Stationarity is often an unrealistic prior assumption for Gaussian process regression. One solution is to predefine an explicit nonstationary covarian...
热度:69
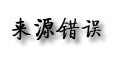
A Decoupled Approach to Exemplar-based Unsupervised Learning[基于范例的无监督学习的解耦方法]
Sebastian Nowozin(马克斯普朗克研究所) A recent trend in exemplar based unsupervised learning is to formulate the learning problem as a convex optimization problem. Convexity is achieved by...
热度:51
Sebastian Nowozin(马克斯普朗克研究所) A recent trend in exemplar based unsupervised learning is to formulate the learning problem as a convex optimization problem. Convexity is achieved by...
热度:51