开课单位--芝加哥丰田技术学院
11
12
13
14
15
16
17
18
19
20
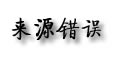
Super-Linear Convergence of Dual Augmented Lagrangian Algorithm for Sparse Learning[稀疏学习的对偶增强拉格朗日算法的超线性收敛性]
Ryota Tomioka(芝加哥丰田技术学院) We analyze the convergence behaviour of a recently proposed algorithm for sparse learning called Dual Augmented Lagrangian (DAL). We theoretically ana...
热度:45
Ryota Tomioka(芝加哥丰田技术学院) We analyze the convergence behaviour of a recently proposed algorithm for sparse learning called Dual Augmented Lagrangian (DAL). We theoretically ana...
热度:45
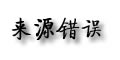
Tutorial on Machine Learning Reductions[关于减少机器学习的教程]
John Langford(芝加哥丰田技术学院) There are several different classification problems commonly encountered in real world applications such as 'importance weighted classification...
热度:38
John Langford(芝加哥丰田技术学院) There are several different classification problems commonly encountered in real world applications such as 'importance weighted classification...
热度:38
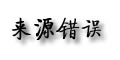
Fast, Exact Nearest Neighbor in Arbitrary Dimensions with a Cover Tree[覆盖树在任意维上的快速精确近邻]
John Langford(芝加哥丰田技术学院) Given only a metric between points, how quickly can the nearest neighbor of a point be found? In the worst case, this time is O(n). When these points ...
热度:57
John Langford(芝加哥丰田技术学院) Given only a metric between points, how quickly can the nearest neighbor of a point be found? In the worst case, this time is O(n). When these points ...
热度:57
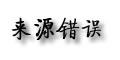
Generalization Bounds and Consistency for Latent Structural Probit and Ramp Loss[潜在结构概率和斜坡损失的概化边界和一致性]
David McAllester(芝加哥丰田技术学院) We consider latent structural versions of probit loss and ramp loss. We show that these surrogate loss functions are consistent in the strong sense th...
热度:71
David McAllester(芝加哥丰田技术学院) We consider latent structural versions of probit loss and ramp loss. We show that these surrogate loss functions are consistent in the strong sense th...
热度:71
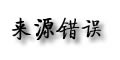
More Data Less Work: Runtime As A Monotonically Decreasing Function of Data Set Size[更多数据更少工作:运行时作为单调减少数据集大小的功能]
Nathan Srebro(芝加哥丰田技术学院) We are used to studying runtime as an increasing function of the data set size, and are happy when this increase is not so bad (e.g. when the runtime ...
热度:72
Nathan Srebro(芝加哥丰田技术学院) We are used to studying runtime as an increasing function of the data set size, and are happy when this increase is not so bad (e.g. when the runtime ...
热度:72
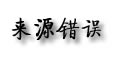
Unsupervised Learning for Stereo Vision [立体视觉的无监督学习]
David McAllester(芝加哥丰田技术学院) We consider the problem of learning to estimate depth from stereo image pairs. This can be formulated as unsupervised learning - the training pairs ar...
热度:75
David McAllester(芝加哥丰田技术学院) We consider the problem of learning to estimate depth from stereo image pairs. This can be formulated as unsupervised learning - the training pairs ar...
热度:75
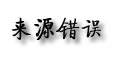
Learning on Structured Data[学习结构化数据]
David McAllester(芝加哥丰田技术学院) Discriminative learning framework is one of the very successful fields of machine learning. The methods of this paradigm, such as Boosting, and Suppor...
热度:63
David McAllester(芝加哥丰田技术学院) Discriminative learning framework is one of the very successful fields of machine learning. The methods of this paradigm, such as Boosting, and Suppor...
热度:63
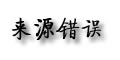
Learning on Structured Data[学习结构化数据]
Yasemin Altun(芝加哥丰田技术学院) Discriminative learning framework is one of the very successful fields of machine learning. The methods of this paradigm, such as Boosting, and Suppor...
热度:23
Yasemin Altun(芝加哥丰田技术学院) Discriminative learning framework is one of the very successful fields of machine learning. The methods of this paradigm, such as Boosting, and Suppor...
热度:23
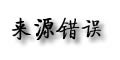
Multiview Clustering via Canonical Correlation Analysis[基于典型相关分析的多视图聚类]
Karen Livescu(芝加哥丰田技术学院) Clustering algorithms such as k-means perform poorly when the data is highdimensional. A number of efficient clustering algorithms developed in recent...
热度:83
Karen Livescu(芝加哥丰田技术学院) Clustering algorithms such as k-means perform poorly when the data is highdimensional. A number of efficient clustering algorithms developed in recent...
热度:83
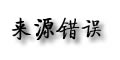
Stochastic Methods for L1 Regularized Loss Minimization[L1正则化损失最小化的随机方法]
Ambuj Tewari(芝加哥丰田技术学院) We describe and analyze two stochastic methods for $\ell_1$ regularized loss minimization problems, such as the Lasso. The first method updates the we...
热度:37
Ambuj Tewari(芝加哥丰田技术学院) We describe and analyze two stochastic methods for $\ell_1$ regularized loss minimization problems, such as the Lasso. The first method updates the we...
热度:37