开课单位--京都大学
1
2
3
4
5
6
7
8
9
10
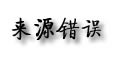
Hierarchically Porous Materials: Preparation and Application to Separation Sciences[分级多孔材料的制备及其在分离科学中的应用]
Kazuki Nakanishi(京都大学) Polymerization-induced phase separation in chemically cross-linked systems has been applied to obtain hierarchically porous monolithic materials in a ...
热度:74
Kazuki Nakanishi(京都大学) Polymerization-induced phase separation in chemically cross-linked systems has been applied to obtain hierarchically porous monolithic materials in a ...
热度:74
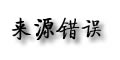
Neural mechanisms of working memory in the prefrontal cortex[在额叶皮层工作记忆的神经机制]
Shintaro Funahashi(京都大学) Working memory is a mechanism for short-term active maintenance of information as well as for processing maintained information. The dorsolateral pref...
热度:153
Shintaro Funahashi(京都大学) Working memory is a mechanism for short-term active maintenance of information as well as for processing maintained information. The dorsolateral pref...
热度:153
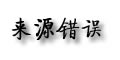
Structural equations and divisive normalization for energy-dependent component analysis[能量相关成分分析的结构方程和分裂归一化]
Jun-ichiro Hirayama(京都大学) Components estimated by independent component analysis and related methods are typically not independent in real data. A very common form of nonlinear...
热度:74
Jun-ichiro Hirayama(京都大学) Components estimated by independent component analysis and related methods are typically not independent in real data. A very common form of nonlinear...
热度:74
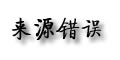
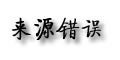
Optimal Online Learning Procedures for Model-Free Policy Evaluation[无模型政策评估的最佳在线学习程序]
Tsuyoshi Ueno(京都大学) In this study, we extend the framework of semiparametric statistical inference introduced recently to reinforcement learning (Ueno, et.al., 2008) to o...
热度:79
Tsuyoshi Ueno(京都大学) In this study, we extend the framework of semiparametric statistical inference introduced recently to reinforcement learning (Ueno, et.al., 2008) to o...
热度:79
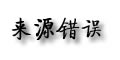
Prefrontal cortex and decision-making[前额叶皮层与决策]
Shintaro Funahashi(京都大学) The prefrontal cortex has been known to participate in working memory processes. Tonic sustained activation observed during the delay period (delay-pe...
热度:130
Shintaro Funahashi(京都大学) The prefrontal cortex has been known to participate in working memory processes. Tonic sustained activation observed during the delay period (delay-pe...
热度:130
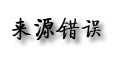
Boosted optimization for network classification[网络分类的优化改进]
Timothy Hancock(京都大学) In this paper we propose a new classification algorithm designed for application on complex networks motivated by algorithmic similarities between boo...
热度:53
Timothy Hancock(京都大学) In this paper we propose a new classification algorithm designed for application on complex networks motivated by algorithmic similarities between boo...
热度:53
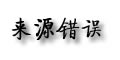
Submodularity and Discrete Convexity[子模性与离散凸性 ]
Satoru Fujishige(京都大学) The present talk is a tutorial one and gives some essence of submodular functions and discrete convexity. The contents of my talk will be the followi...
热度:262
Satoru Fujishige(京都大学) The present talk is a tutorial one and gives some essence of submodular functions and discrete convexity. The contents of my talk will be the followi...
热度:262
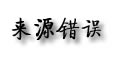
Kernels on histograms through the transportation polytope[通过运输多面体的直方图上的内核]
Marco Cuturi(京都大学) For two integral histograms and of equal sum, the Monge-Kantorovich distance MK(r,c) between r and c parameterized by a d × d cost matrix T is the min...
热度:84
Marco Cuturi(京都大学) For two integral histograms and of equal sum, the Monge-Kantorovich distance MK(r,c) between r and c parameterized by a d × d cost matrix T is the min...
热度:84
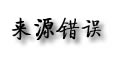
A Spectral Clustering Approach to Optimally Combining Numerical Vectors with a Modular Network[数字矢量与模块化网络优化组合的谱聚类方法 ]
Motoki Shiga(京都大学 ) We address the issue of clustering numerical vectors with a network. The problem setting is basically equivalent to constrained clustering by Wagstaff...
热度:60
Motoki Shiga(京都大学 ) We address the issue of clustering numerical vectors with a network. The problem setting is basically equivalent to constrained clustering by Wagstaff...
热度:60